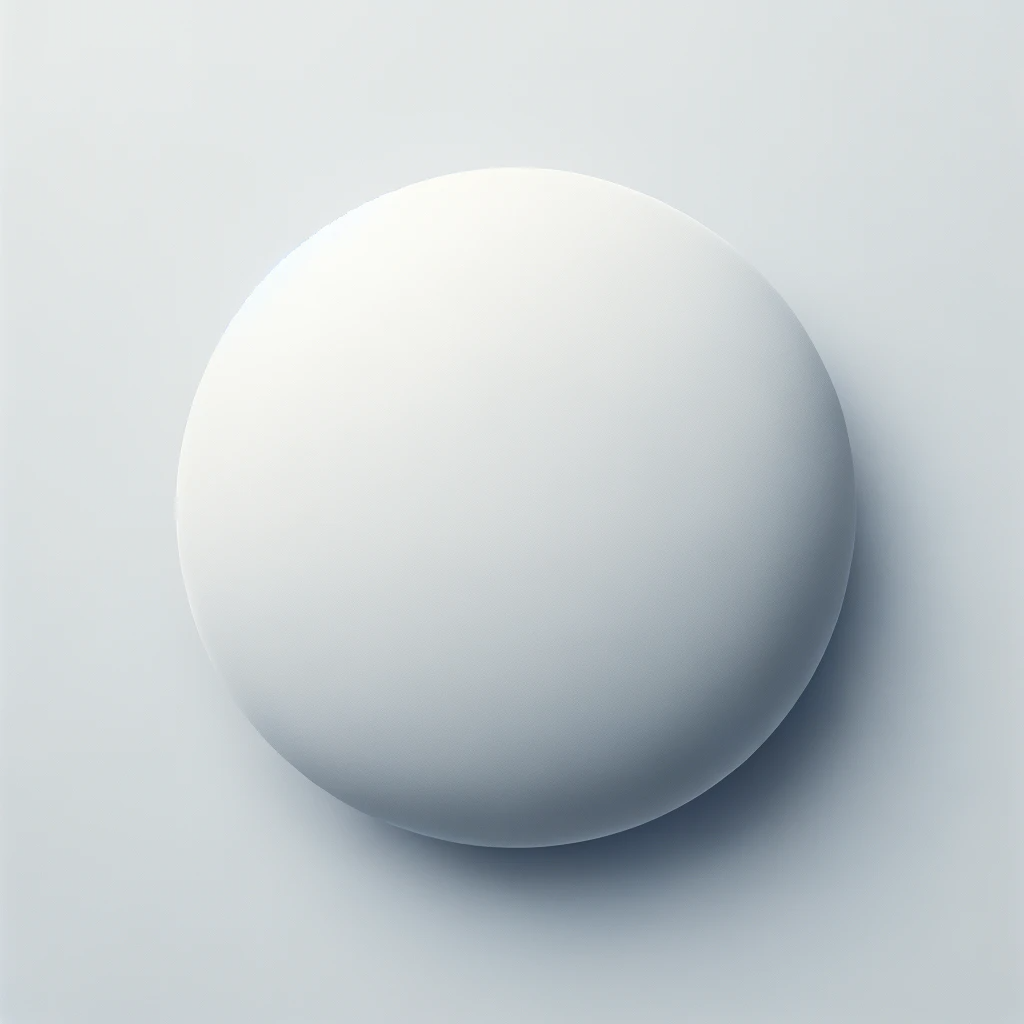
Abstract. Machine learning techniques such as classification and regression trees (CART) have been suggested as promising alternatives to logistic regression for the estimation of propensity scores. The authors examined the performance of various CART-based propensity score models using simulated data. Hypothetical studies of varying …Using Propensity Modeling to Drive Revenue and Increase Engagement. Here's a quick overview of what you need to know about propensity modeling and how it can help your association.If the model predicts a treatment propensity of 80%, the true probability should also be 80%. A calibration plot visualizes this, as shown below. If the calibration plot shows (vertical) errors ...Nov 22, 2014 · This class of predictive analytics is called buyer propensity models or customer targeting models. With this approach, you build models that predict the likelihood that a prospective customer will respond to your mailing campaign. In this chapter, we will show you how to build this class of models in Azure Machine Learning. Propensity modeling uses machine learning and AI to analyze millions of data sets and predict whether someone will take a particular action, such as making a purchase or churning. This allows marketers to identify the highest value consumer (those most likely to take the desired action) and focus their efforts on reaching them with the …Jun 27, 2020 · Traditional Propensity Model. This is actually not an uplift model but needs to be included because it is the classic approach to the uplift problem. The algorithm will typically be a classifier like logistic regression. These algorithms can output a probability 0 to 1 that can be used to sort the audience. Propensity modeling and predictive analysis are methods of assessing what action a customer is likely to take based on available data. This can help lenders identify “trigger” events likely to indicate a prospect on the market for a loan. For example, historical data indicates that when a person gets married, they typically buy a house ... In this review, we discuss critical aspects in the use of propensity scores in pharmacoepidemiologic research. We address study design, covariate choice, model selection, using the propensity score, and strategies for dealing with unmeasured bias. For each, we highlight current understanding, recent developments, and opportunities for …Propensity Score Matching (PSM) is a quasi-experimental method in which the researcher uses statistical techniques to construct an artificial control group by matching each treated unit with a non-treated unit of similar characteristics. Using these matches, the researcher can estimate the impact of an intervention.Are you interested in exploring the world of 3D modeling but don’t want to invest in expensive software? Luckily, there are several free 3D modeling software options available that...You can test drive it for 1,000 miles with a full refund. Tesla announced its long-awaited $35,000 Model 3 today (Feb. 28). For more than two years, Tesla has been ramping up produ...Aug 29, 2022 · Propensity modeling is a cycli cal process and continuous journey. That is, the model improves over time as more data is gathered, allowing you to hone in on which targeted outreach strategy works best for each member, attendee, donor, subscriber, or audience in question. Chevrolet car models come in all shapes and price ranges. See pictures and learn about the specs, features and history of Chevrolet car models. Advertisement Chevrolet has been a c...Aug 18, 2020 ... Propensity modelling is a process of assigning propbabilities to commit a certain action (e.g. to buy, to churn, etc.) ...Nov 27, 2023 · Propensity Modeling. Propensity Modeling, on the other hand, focuses on predicting the likelihood of a specific event or behavior occurring. It involves assigning a probability score to each individual, indicating the likelihood of them taking a desired action, such as making a purchase or clicking on an ad. Applications: In the statistical analysis of observational data, propensity score is a technique that attempts to estimate the effect of a treatment (exposure) by accounting for the covariates that predict ...In this review, we discuss critical aspects in the use of propensity scores in pharmacoepidemiologic research. We address study design, covariate choice, model selection, using the propensity score, and strategies for dealing with unmeasured bias. For each, we highlight current understanding, recent developments, and opportunities for …Aug 3, 2022 · A propensity model uses machine learning to analyze your sales data and pinpoint which past prospects have purchased from you, and which have not, even …Model trains are a popular hobby for many people, and O scale model trains are some of the most popular. O scale model trains are a great way to get started in the hobby, as they a...Jul 19, 2019 · Propensity modeling becomes a lifecycle when you use follow-up testing to better define your targets and goals. Once the analytics (models) are deployed, a few follow-up validation practices are suggested: Utilize testing (e.g., messaging, creative) Profile and segment campaign responders, as well as campaign converters.Aug 1, 2023 · Propensity Model Deployment. To address this issue, the company deployed a logistic regression model that incorporated CRM and transaction data. The CRM data included variables such as time since ...Jul 19, 2019 · Propensity modeling becomes a lifecycle when you use follow-up testing to better define your targets and goals. Once the analytics (models) are deployed, a few follow-up validation practices are suggested: Utilize testing (e.g., messaging, creative) Profile and segment campaign responders, as well as campaign converters.The model's probabilistic estimate that a user will start drinking Soylent is called a propensity score. Form some number of buckets, say 10 buckets in total (one bucket covers users with a 0.0 - 0.1 propensity to take the drink, a second bucket covers users with a 0.1 - 0.2 propensity, and so on), and place people into each one.The binomial model is an options pricing model. Options pricing models use mathematical formulae and a variety of variables to predict potential future prices of commodities such a...Building a propensity model involves five steps. We will cover the process end to end. If you are interested primarily in technical topics, such as algorithm selection, go straight to Step 4. 1. STEP 1: Create a dataset. Compile an aggregated dataset ready to use by your model. 2. STEP 2: Create a model. Create a … See moreMar 7, 2022 · Examples of propensity modeling include predicting which consumers are likely to buy a product, to sign up for a service, or even to churn and no longer be an active customer for a brand. The output of a propensity model is a score between 0 and 1 for each consumer, where this score represents how likely the consumer is to take that action. In general, poor excellent discrimination, meaning that 90% of the model fit is a function of problems related to model time the propensity score of a treated individual was development, such as continuous variable misspeci- higher when compared to the propensity score of an fication, inappropriate interaction terms or omitted untreated …Feb 11, 2021. 2. Photo by Nadin Mario on Unsplash. This is a joint work with Aleix Ruiz, Jesús Cerquides, Joan Capdevila and Borja Velasco within the Causal ALGO Bcn. You … This blog will provide 1) a primer on propensity modeling, 2) types of lead scoring, 3) some finer details on the modeling process, 4) testing a model for effectiveness, and 5) implementing a model. Propensity Modeling. Propensity modeling is predicting the likelihood – or propensity – of a lead, person, or target company to convert on your ... Nov 20, 2023 · Model 8: Propensity to convert. The propensity-to-convert model can predict the likelihood that a customer will accept your offer. This model can be used for direct mail campaigns where the cost of marketing is high, for instance. In this case, you only want to send the offers to customers with a high propensity to convert. : Modeling the Risk Propensity Scale by confirmatory factor analysis : SEM factor loadings "now" and "past" Risk Propensity Scale items on domains, and of overall risk propensity on each domainPropensity modeling is the action of defining consumer segments with strong likelihoods of converting. Propensity marketing is the strategy of reaching and engaging with those identified consumers. How Does Propensity Marketing Differ From Lookalike Marketing?A person’s attitudes and behaviors, as well as a propensity for certain health conditions, are often part of the nature versus nurture debate. The roles of a person’s chemical make...Feb 11, 2021 · Computing the propensity score is relatively simple, even in high dimensional sets of covariates. In these cases what we could do is model it using logistic regression with treatment as the target variable. But to be able to use this propensity score in the methods we will review next, there are some constraints. Using Propensity Modeling to Drive Revenue and Increase Engagement. Here's a quick overview of what you need to know about propensity modeling and how it can help your association.In a report released today, Matthew VanVliet from BTIG reiterated a Buy rating on Model N (MODN – Research Report), with a price target of... In a report released today, Matt...Model trains are a great hobby for people of all ages. O scale model trains are one of the most popular sizes and offer a wide variety of options for both experienced and novice mo... Propensity Score Analysis of Complex Survey Data with Structural Equation Modeling: A Tutorial with Mplus. Structural Equation Modeling : A Multidisciplinary Journal , 26:3, 448-469, DOI: 10.1080/10705511.2018.1522591 The Tesla Model 3 is one of the most advanced electric cars on the market today. It’s a sleek, stylish, and efficient vehicle that has revolutionized the way we think about electri...Aug 5, 2021 · The PS methods’ primary modeling decisions are for a propensity model. Although the propensity models and outcome models conditional on measured covariates are both prone to misspecification, one may feel more confident of correctly specifying an exposure model in situations where more knowledge about the relationships with covariates is ... Nov 15, 2022 · Propensity Modeling is a powerful #dataanalytics tool to help segment your customers. This video explores what is propensity modeling, what are some types of...In This Master Class, We Partnered With TCV, A Growth Equity Firm That Provides Management Teams Of Growth Stage Companies With Data-Driven Insights And ...Photo by Bruna Branco on Unsplash. In this post I estimate a number of models and assess their performance and fit to the data using a model-agnostic methodology that enables to compare ...May 17, 2023 · A propensi ty score is the probability that an individual will be exposed to the treatment. If we took 100 identical customers and 70 of them were exposed to the treatment then they would all have a propensity score of 0.7. The most common approach to calculating the propensity score is to fit a logistic regression classifier to predict the ... Propensity modeling, often referred to as predictive modeling or propensity scoring, serves as a statistical technique designed to forecast the likelihood of an individual or group engaging in a ... Model inputs. To generate values, the Churn Propensity model uses the following data. Note : When you select Custom - Zero orders in the Churn criteria parameter, the algorithm auto-calculates the churn output values (ChurnScore and ChurnRisk). For the model to successfully run: Data needs to be ingested into all the input attributes below. Feb 17, 2023 · Propensity model data set. | Screenshot: Zolzaya Luvsandorj. We didn’t partition the data into a train and test split because we aren’t building a predictive model. Propensity score tells us the probability of an individual getting the treatment given the confounders. We have also prepared the logit transformation of the propensity score.Oct 7, 2021 · Learn how to use different propensity modeling techniques, such as logistic regression, random forest, and XGBoost, to guide A/B tests and improve digital …Jun 21, 2022 · The key is to understand the goals, goal values and the data points necessary to make the model work best. 2. Know data attributes. Propensity models need good data to provide the best insights. The more pertinent data points the model can use, the more likely the web personalization will achieve the desired goals. A TWO-STEP BAYESIAN PROPENSITY SCORE ANALYSIS. As noted earlier, a recent paper by Kaplan and Chen (2012) advanced a two-step approach to Bayesian propensity score analysis that was found to quite accurately estimate the treatment effect while at the same time preventing undesirable feedback between the propensity score model and …Oct 23, 2019 · Other approaches to estimate the propensity score—for instance, the covariate balancing propensity scores or machine learning approaches such as neural networks—could provide alternatives that are less prone to misspecification.20 21 Regardless of the approach used for constructing propensity score models, researchers should emphasise inclusion of …Apr 19, 2021 · A propensity model is a statistical analysis of your target audience that predicts their future actions, using a range of datasets that typically includes demographics, psychographics (eg: interests) and previous behaviour. For example, you could create a propensity model for existing customers using their personal data, purchase history and …For example, one review found that of 45 propensity score applications including linear predictors of treatment and using logistic regression, only one reported an assessment of the assumption of linearity in the logit . The same review found that use of interaction terms in propensity score models was infrequent at best .Propensity Model Performance Evaluation – Model Training Performance. The standard way to evaluate a machine learning model is to divide your data randomly into ...Propensity modeling isn't always a walk in the park, particularly with legacy systems that need to be revamped in order to support advanced artificial intelligence features. However, with careful planning and execution, CIOs can overcome these challenges and reap the benefits of propensity models in their organizations.Jul 28, 2023 · A propensity model is a statistical approach used to predict the likelihood of a specific event occurring based on historical data. By analyzing past behaviors and …Mar 19, 2024 · November 10, 2022. How to Build a Propensity Model. Written by: Max Malacari. Commercial teams often use historical data to measure performance, assess …A TWO-STEP BAYESIAN PROPENSITY SCORE ANALYSIS. As noted earlier, a recent paper by Kaplan and Chen (2012) advanced a two-step approach to Bayesian propensity score analysis that was found to quite accurately estimate the treatment effect while at the same time preventing undesirable feedback between the propensity score model and …Oct 23, 2019 · Propensity scores1 have become a cornerstone of confounding adjustment in observational studies evaluating outcomes of treatment use in routine care. Propensity score based methods target causal inference in observational studies in a manner similar to randomised experiments by facilitating the measurement of differences in outcomes between the treated population and a reference population.2 ... Nov 10, 2016 · Essentially, propensity modeling correlates customer characteristics with anticipated behaviors or propensities. It tracks buying habits as well as other actions such as a customer’s propensity to open a marketing email, sign up to a loyalty program, or participate in feedback surveys. Its success is underpinned by the quality of your ... . Deliver personalized customer experiences · Enable the persisPurpose: To document which established criteria for logi Jul 28, 2023 · A propensity model is a statistical approach used to predict the likelihood of a specific event occurring based on historical data. By analyzing past behaviors and … Mar 7, 2022 · Examples of prope Model inputs. To generate values, the Churn Propensity model uses the following data. Note : When you select Custom - Zero orders in the Churn criteria parameter, the algorithm auto-calculates the churn output values (ChurnScore and ChurnRisk). For the model to successfully run: Data needs to be ingested into all the input attributes below. Jun 20, 2023 · What is Propensity Modeling. In simple terms, propensity modeling is like a crystal ball for businesses. It’s a tool that uses past data and statistics to predict how … Dec 28, 2022 ... Description ... Buyer pr...
Continue Reading