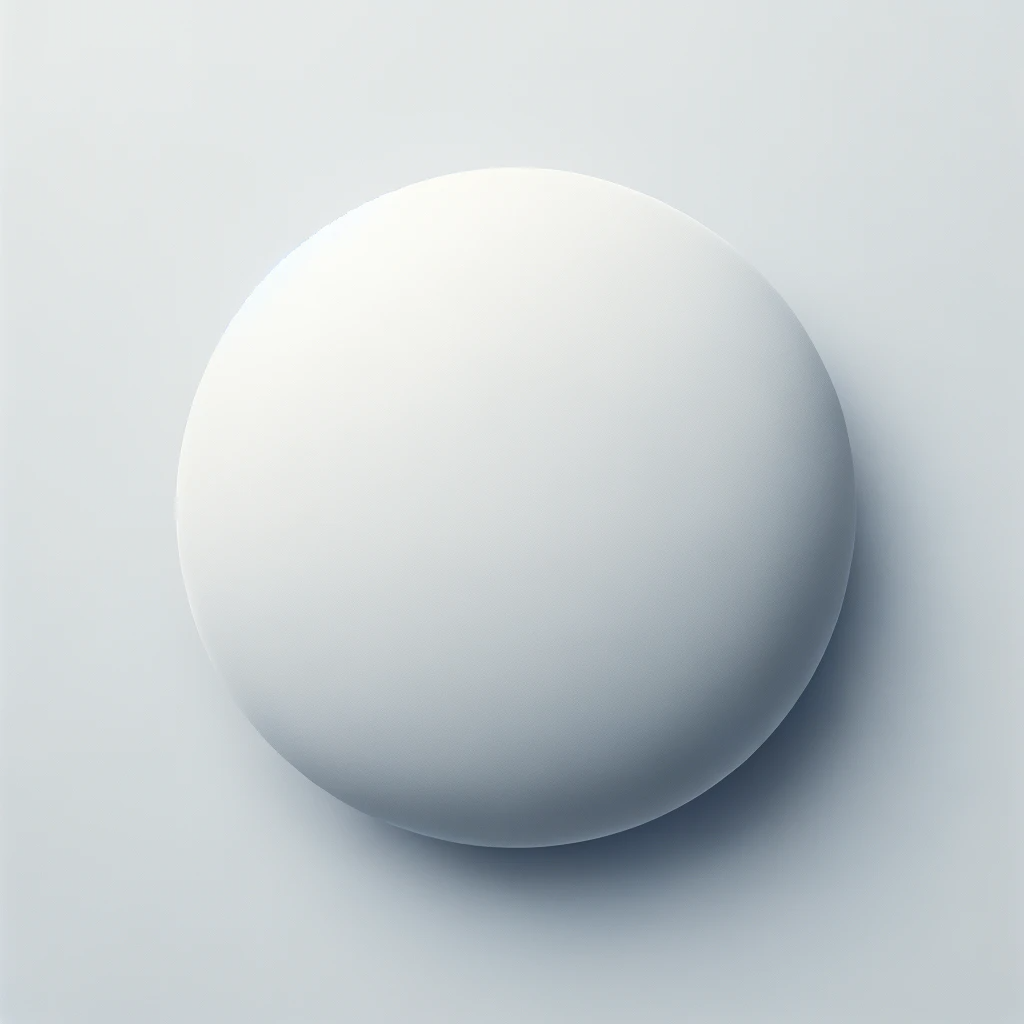
Introduction. Distributed Random Forest (DRF) is a powerful classification and regression tool. When given a set of data, DRF generates a forest of classification or regression trees, rather than a single classification or regression tree. Each of these trees is a weak learner built on a subset of rows and columns.The Cricut Explore Air 2 is a versatile cutting machine that allows you to create intricate designs and crafts with ease. To truly unlock its full potential, it’s important to have... ランダムフォレスト. ランダムフォレスト ( 英: random forest, randomized trees )は、2001年に レオ・ブレイマン ( 英語版 ) によって提案された [1] 機械学習 の アルゴリズム であり、 分類 、 回帰 、 クラスタリング に用いられる。. 決定木 を弱学習器とする ... 24 Dec 2021 ... I have seen some jaw-dropping examples of neural networks and deep learning (e.g., deep fakes). I am looking for similarly awesome examples of ...Step 1: Select n (e.g. 1000) random subsets from the training set. Step 2: Train n (e.g. 1000) decision trees. one random subset is used to train one decision tree; the optimal splits for each decision tree are based on a random subset of features (e.g. 10 features in total, randomly select 5 out of 10 features to split)Random forests are a combination of tree predictors such that each tree depends on the values of a random vector sampled independently and with the same distribution for all trees in the forest ... Machine Learning, 36(1/2), 105-139. Google Scholar Digital Library; Breiman, L. (1996a). Bagging predictors. Machine Learning …Une Random Forest (ou Forêt d’arbres de décision en français) est une technique de Machine Learning très populaire auprès des Data Scientists et pour cause : elle présente de nombreux avantages comparé aux autres algorithmes de data. C’est une technique facile à interpréter, stable, qui présente en général de bonnes accuracies ...30 Jan 2019 ... 1 Answer 1 ... Your problem is not with the model but with the underlying concept. A model needs to learn to generate good features. You are ...Michaels is an art and crafts shop with a presence in North America. The company has been incredibly successful and its brand has gained recognition as a leader in the space. Micha...Model Development The proposed model was built using the random forest algorithm. The random forest was implemented using the RandomForestClassifier available in Phyton Scikit-learn (sklearn) machine learning library. Random Forest is a popular supervised classification and regression machine learning technique.As technology becomes increasingly prevalent in our daily lives, it’s more important than ever to engage children in outdoor education. PLT was created in 1976 by the American Fore...10 Mar 2022 ... Comments39 · Feature selection in Machine Learning | Feature Selection Techniques with Examples | Edureka · Random Forest Algorithm - Random ...Random Forests is a Machine Learning algorithm that tackles one of the biggest problems with Decision Trees: variance. Even though Decision Trees is simple …May 12, 2021 · Machine learning algorithms, particularly Random Forest, can be effectively used in long-term outcome prediction of mortality and morbidity of stroke patients. NIHSS at 24, 48 h and axillary ... Apr 14, 2021 · The entire random forest algorithm is built on top of weak learners (decision trees), giving you the analogy of using trees to make a forest. The term “random” indicates that each decision tree is built with a random subset of data. Here’s an excellent image comparing decision trees and random forests: Random Forest in Machine Learning is a method for classification (classifying an experiment to a category), or regression (predicting the outcome of an experiment), based on the training data (knowledge of previous experiments). Random forest handles non-linearity by exploiting correlation between the features of data-point/experiment. Random forests or random decision forests is an ensemble learning method for classification, regression and other tasks that operates by constructing a multitude of decision trees at training time. For classification tasks, the output of the random forest is the class selected by most trees. Random forests perform better than a single decision tree for a wide range of data items. Even when a major amount of the data is missing, the Random Forest algorithms maintain high accuracy. Features of Random Forest in Machine Learning. Following are the major features of the Random Forest Algorithm –The probabilistic mapping of landslide occurrence at a high spatial resolution and over a large geographic extent is explored using random forests (RF) machine learning; light detection and ranging (LiDAR)-derived terrain variables; additional variables relating to lithology, soils, distance to roads and streams and cost distance to roads and streams; …Random Forest is a powerful and versatile supervised machine learning algorithm that grows and combines multiple decision trees to create a “forest.” It can be used for both classification and …A famous machine learning classifier Random Forest is used to classify the sentences. It showed 80.15%, 76.88%, and 64.41% accuracy for unigram, bigram, and trigram features, respectively.Random Forest is a popular machine learning algorithm that belongs to the supervised learning technique. It can be used for both Classification and Regression problems in …High-speed railways (HSRs) are established all over the world owing to their advantages of high speed, ride comfort, and low vibration and noise. A ballastless track slab is a crucial part of the HSR, and its working condition directly affects the safe operation of the train. With increasing train operation time, track slabs suffer from various defects …Non-clinical approaches like machine learning, data mining, deep learning, and other artificial intelligence approaches are among the most promising approaches for use outside of a clinical setting. ... Based on the success evaluation, the Random Forest had the best precision of 94.99%. Published in: 2021 12th International Conference on ...23 Dec 2018 ... Random forest is a popular regression and classification algorithm. In this tutorial we will see how it works for classification problem in ... Random Forest is a popular machine learning algorithm that belongs to the supervised learning technique. It can be used for both Classification and Regression problems in ML. It is based on the concept of ensemble learning, which is a process of combining multiple classifiers to solve a complex problem and to improve the performance of the model. Random Forests. Random forests (RF) construct many individual decision trees at training. Predictions from all trees are pooled to make the final prediction; the mode of the classes for classification or the mean prediction for regression. As they use a collection of results to make a final decision, they are referred to as Ensemble techniques.A random forest trains each decision tree with a different subset of training data. Each node of each decision tree is split using a randomly selected attribute from the data. This element of randomness ensures that the Machine Learning algorithm creates models that are not correlated with one another.Random forests are one the most popular machine learning algorithms. They are so successful because they provide in general a good predictive performance, low overfitting, and easy interpretability. This interpretability is given by the fact that it is straightforward to derive the importance of each variable on the tree decision.The Random Forest algorithm comes along with the concept of Out-of-Bag Score (OOB_Score). Random Forest, is a powerful ensemble technique for machine learning and data science, but most people tend to skip the concept of OOB_Score while learning about the algorithm and hence fail to understand the complete importance of …Are you someone who is intrigued by the world of data science? Do you want to dive deep into the realm of algorithms, statistics, and machine learning? If so, then a data science f...Machine learning projects have become increasingly popular in recent years, as businesses and individuals alike recognize the potential of this powerful technology. However, gettin...Machine learning methods, such as random forest, artificial neural network, and extreme gradient boosting, were tested with feature selection techniques, including feature importance and principal component analysis. The optimal combination was found to be the XGBoost method with features selected by PCA, which outperformed other …One of the biggest machine learning events is taking place in Las Vegas just before summer, Machine Learning Week 2020 This five-day event will have 5 conferences, 8 tracks, 10 wor...Large Hydraulic Machines - Large hydraulic machines are capable of lifting and moving tremendous loads. Learn about large hydraulic machines and why tracks are used on excavators. ...Random Forest. bookmark_border. This is an Ox. Figure 19. An ox. In 1906, a weight judging competition was held in England . 787 participants guessed the weight …We selected the random forest as the machine learning method for this study as it has been shown to outperform traditional regression. 15 It is a supervised machine learning approach known to extract information from noisy input data and learn highly nonlinear relationships between input and target variables. Random forest …Apr 14, 2021 · The entire random forest algorithm is built on top of weak learners (decision trees), giving you the analogy of using trees to make a forest. The term “random” indicates that each decision tree is built with a random subset of data. Here’s an excellent image comparing decision trees and random forests: 24 Dec 2021 ... I have seen some jaw-dropping examples of neural networks and deep learning (e.g., deep fakes). I am looking for similarly awesome examples of ...A Random Forest Algorithm is a supervised machine learning algorithm that is extremely popular and is used for Classification and Regression problems in Machine Learning. We know that a forest comprises numerous trees, and …Introduction. Machine learning algorithms are increasingly being applied in image analysis problems ranging from face recognition to self-driving vehicles .Recently, the Random Forest algorithm , has been used in global tropical forest carbon mapping .However, there is considerable resistance to the use of machine learning algorithms in …Random forests are a popular supervised machine learning algorithm. Random forests are for supervised machine learning, where there is a labeled target variable. Random forests can be used for solving …Conservation machine learning conserves models across runs, users, and experiments—and puts them to good use. We have previously shown the merit of this idea through a small-scale preliminary ...Sep 21, 2023 · Random forests. A random forest ( RF) is an ensemble of decision trees in which each decision tree is trained with a specific random noise. Random forests are the most popular form of decision tree ensemble. This unit discusses several techniques for creating independent decision trees to improve the odds of building an effective random forest. Abstract. Random forests are a combination of tree predictors such that each tree depends on the values of a random vector sampled independently and with the same distribution …Apr 14, 2021 · The entire random forest algorithm is built on top of weak learners (decision trees), giving you the analogy of using trees to make a forest. The term “random” indicates that each decision tree is built with a random subset of data. Here’s an excellent image comparing decision trees and random forests: Machine learning algorithms are at the heart of predictive analytics. These algorithms enable computers to learn from data and make accurate predictions or decisions without being ...Out of bag (OOB) score is a way of validating the Random forest model. Below is a simple intuition of how is it calculated followed by a description of how it is different from validation score and where it is advantageous. For the description of OOB score calculation, let’s assume there are five DTs in the random forest ensemble … A 30-m Landsat-derived cropland extent product of Australia and China using random forest machine learning algorithm on Google Earth Engine cloud computing platform. ISPRS J. Photogramm. Remote Sens. 2018, 144, 325–340. [Google Scholar] Pal, M. Random forest classifier for remote sensing classification. Int. J. Remote Sens. 2005, 26, 217–222 18 Aug 2020 ... Space and time complexity of the decision tree model is relatively higher, leading to longer model training time. A single decision tree is ...RAPIDS’s machine learning algorithms and mathematical primitives follow a familiar scikit-learn-like API. Popular tools like XGBoost, Random Forest, and many others are supported for both single-GPU and large data center deployments. For large datasets, these GPU-based implementations can complete 10-50X faster than their CPU equivalents.Aboveground biomass (AGB) is a fundamental indicator of forest ecosystem productivity and health and hence plays an essential role in evaluating forest carbon reserves and supporting the development of targeted forest management plans. Here, we proposed a random forest/co-kriging framework that integrates the strengths of …Sep 28, 2019 · Random Forest = Bagging + Decision Tree. 步驟. 定義大小為n的隨機樣本(這裡指的是用bagging方法),就是從資料集中隨機選取n個資料,取完後放回。. 從選取 ... Accordingly, the goal of this thesis is to provide an in-depth analysis of random forests, consistently calling into question each and every part of the algorithm, in order to shed new light on its learning capabilities, inner workings and interpretability. The first part of this work studies the induction of decision trees and the construction ...Machine learning for prediction of all-cause mortality in patients with suspected coronary artery disease: a 5-year multicentre prospective registry analysis: 257 : Random forest-based similarity measures for multi-modal classification of Alzheimer’s disease: 248 : Effective Heart disease prediction Using hybrid Machine Learning …Whenever you think of data science and machine learning, the only two programming languages that pop up on your mind are Python and R. But, the question arises, what if the develop...The Random Forest algorithm forms part of a family of ensemble machine learning algorithms and is a popular variation of bagged decision trees. It also comes implemented in the OpenCV library. In this tutorial, you will learn how to apply OpenCV's Random Forest algorithm for image classification, starting with a relatively easier …14 May 2023 ... Intellipaat's Advanced Certification in Data Science and AI: ...In summary, here are 10 of our most popular random forest courses. Machine Learning: DeepLearning.AI. Advanced Learning Algorithms: DeepLearning.AI. Predict Ideal Diamonds over Good Diamonds using a Random Forest using R: Coursera Project Network. Neural Networks and Random Forests: LearnQuest.Whenever you think of data science and machine learning, the only two programming languages that pop up on your mind are Python and R. But, the question arises, what if the develop...Random forest is an ensemble machine learning technique that averages several decision trees on different parts of the same training set, with the objective of overcoming the overfitting problem of the individual decision trees. In other words, a random forest algorithm is used for both classification and regression problem statements that ...If you’re itching to learn quilting, it helps to know the specialty supplies and tools that make the craft easier. One major tool, a quilting machine, is a helpful investment if yo...Feb 7, 2023 · In classical Machine Learning, Random Forests have been a silver bullet type of model. The model is great for a few reasons: Requires less preprocessing of data compared to many other algorithms, which makes it easy to set up; Acts as either a classification or regression model; Less prone to overfitting; Easily can compute feature importance Random forest improves on bagging because it decorrelates the trees with the introduction of splitting on a random subset of features. This means that at each split of the tree, the model considers only a small subset of features rather than all of the features of the model. That is, from the set of available features n, a subset of m features ...Random forest regression is an ensemble learning technique that integrates predictions from various machine learning algorithms to produce more precise predictions than a single model . The proposed random forest technique does not require extensive data preprocessing or imputation of missing values prior to training.A Random Forest Algorithm is a supervised machine learning algorithm that is extremely popular and is used for Classification and Regression problems in Machine Learning. We know that a forest comprises numerous trees, and …Non-clinical approaches like machine learning, data mining, deep learning, and other artificial intelligence approaches are among the most promising approaches for use outside of a clinical setting. ... Based on the success evaluation, the Random Forest had the best precision of 94.99%. Published in: 2021 12th International Conference on ...Random forest is an extension of bagging that also randomly selects subsets of features used in each data sample. Both bagging and random forests have proven effective on a wide range of different predictive modeling problems. ... Bootstrap Aggregation, or Bagging for short, is an ensemble machine learning algorithm.Clustering. What is a random forest. A random forest consists of multiple random decision trees. Two types of randomnesses are built into the trees. First, each tree is built on a random sample from the …Random forest improves on bagging because it decorrelates the trees with the introduction of splitting on a random subset of features. This means that at each split of the tree, the model considers only a small subset of features rather than all of the features of the model. That is, from the set of available features n, a subset of m features ...This is done as a step within the Random forest model algorithm. Random forest creates bootstrap samples and across observations and for each fitted decision tree a random subsample of the covariates/features/columns are used in the fitting process. The selection of each covariate is done with uniform probability in the original bootstrap paper.Sep 28, 2019 · Random Forest = Bagging + Decision Tree. 步驟. 定義大小為n的隨機樣本(這裡指的是用bagging方法),就是從資料集中隨機選取n個資料,取完後放回。. 從選取 ... Machine Learning, 45, 5–32, 2001 c 2001 Kluwer Academic Publishers. Manufactured in The Netherlands. Random Forests LEO BREIMAN Statistics Department, University of California, Berkeley, CA 94720 Editor: Robert E. Schapire Abstract. Random forests are a combination of tree predictors such that each tree depends on the values of a23 Dec 2018 ... Random forest is a popular regression and classification algorithm. In this tutorial we will see how it works for classification problem in ...Random forest is a flexible, easy-to-use supervised machine learning algorithm that falls under the Ensemble learning approach. It strategically combines multiple decision trees (a.k.a. weak learners) to solve a particular computational problem. If we talk about all the ensemble approaches in machine learning, the two most popular ensemble ...The part must be crucial if the assembly fails catastrophically. The parts must not be very crucial if you can't tell the difference after the machine has been created. 26.Give some reasons to choose Random Forests over Neural Networks. In terms of processing cost, Random Forest is less expensive than neural networks.Dec 18, 2017 · A random forest trains each decision tree with a different subset of training data. Each node of each decision tree is split using a randomly selected attribute from the data. This element of randomness ensures that the Machine Learning algorithm creates models that are not correlated with one another. By using a Random Forest (RF) machine learning tool, we train the vegetation reconstruction with available biomized pollen data of present and past conditions to produce broad-scale vegetation patterns for the preindustrial (PI), the mid-Holocene (MH, ∼6,000 years ago), and the Last Glacial Maximum (LGM, ∼21,000 years ago). ...Using Scikit-Learn’s RandomizedSearchCV method, we can define a grid of hyperparameter ranges, and randomly sample from the grid, performing K-Fold CV with each combination of values. As a brief recap before we get into model tuning, we are dealing with a supervised regression machine learning problem.Random forest is an ensemble machine learning algorithm. It is perhaps the most popular and widely used machine learning algorithm given its good or …Aug 31, 2023 · 6. Key takeaways. So there you have it: A complete introduction to Random Forest. To recap: Random Forest is a supervised machine learning algorithm made up of decision trees. Random Forest is used for both classification and regression—for example, classifying whether an email is “spam” or “not spam”. Random Forest is a technique of Machine Learning while Neural Networks are exclusive to Deep Learning. What are Neural Networks? ... Neural nets are another means of machine learning in which a computer learns to perform a task by analyzing training examples. As the neural net is loosely based on the human brain, it will consist …Sep 21, 2023 · Random forests. A random forest ( RF) is an ensemble of decision trees in which each decision tree is trained with a specific random noise. Random forests are the most popular form of decision tree ensemble. This unit discusses several techniques for creating independent decision trees to improve the odds of building an effective random forest. Here, I've explained the Random Forest Algorithm with visualizations. You'll also learn why the random forest is more robust than decision trees.#machinelear...Random Forest is a popular machine learning algorithm that belongs to the supervised learning technique. It can be used for both Classification and Regression problems in …. 30 Jan 2019 ... 1 Answer 1 ... Your problem is not with the mClassification and Regression Tree (CART) is a pred Introduction. Distributed Random Forest (DRF) is a powerful classification and regression tool. When given a set of data, DRF generates a forest of classification or regression trees, rather than a single classification or regression tree. Each of these trees is a weak learner built on a subset of rows and columns.21 Feb 2024 ... Gradient Boosting is defined as a machine learning technique to build predictive models in stages by merging the strengths of weak learners ( ... In this paper, a novel random forest (RF)-based multi Random Forest is a machine learning algorithm used for regression and classification tasks. It is used to identify GWP zones at the downstream part of Wadi Yalamlam. A Random Forest algorithm works by creating multiple decision trees, each of which used a random subset of the explanatory variables, and then averaging their …Machine learning algorithms are at the heart of many data-driven solutions. They enable computers to learn from data and make predictions or decisions without being explicitly prog... Random Forest in Machine Learning is a method for classifica...
Continue Reading