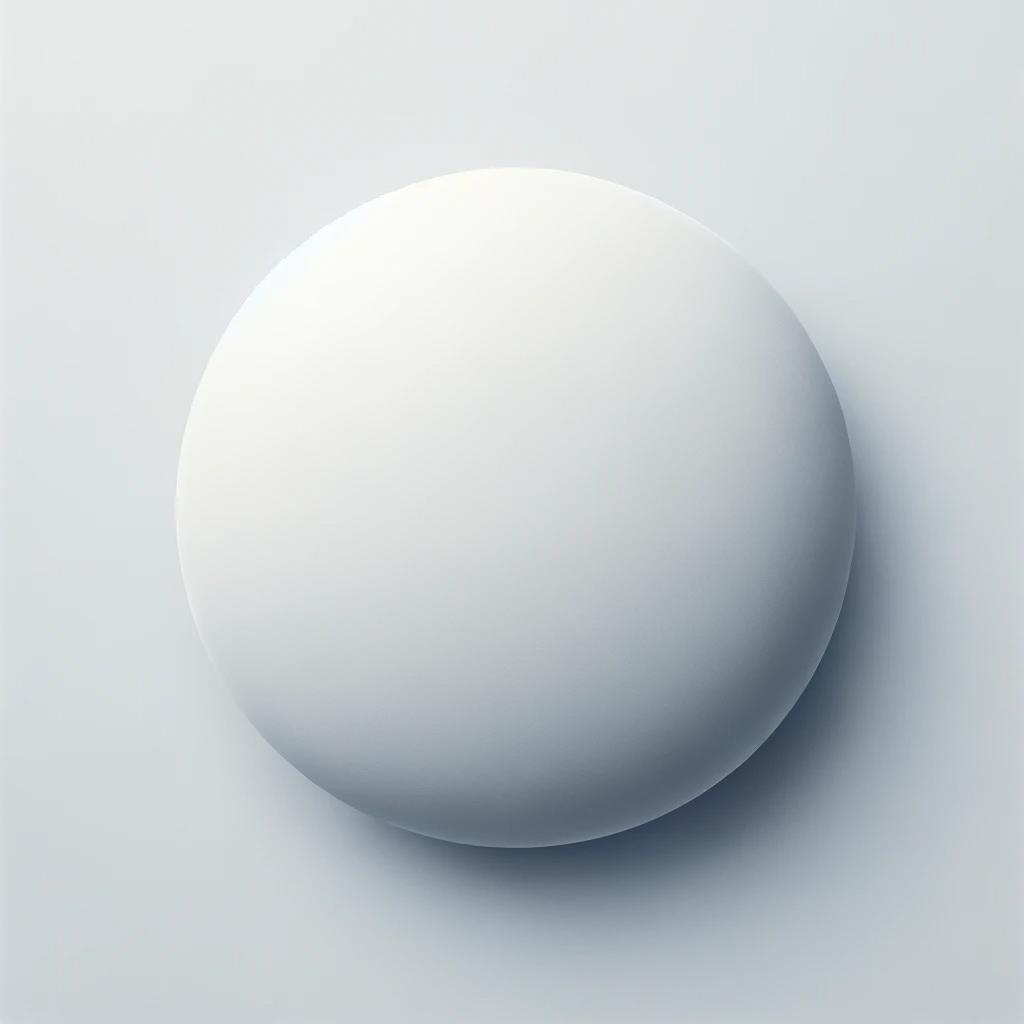
There are two common unsupervised ways to build tasks from the auxiliary dataset: 1) CSS-based methods (Comparative Self-Supervised, as shown in Fig. 1(c)) use data augmentations to obtain another view of the images to construct the image pairs, and then use the image pairs to build tasks [17, 20]; 2) Clustering-based methods (as shown …Unlike unsupervised methods, CellAssign and Garnett require the user to provide a list of marker genes for each cluster. At first, it may seem as if this requirement makes the methods less user ...We have made a first introduction to unsupervised learning and the main clustering algorithms. In the next article we will walk …In this paper, we propose a new distance metric for the K-means clustering algorithm. Applying this metric in clustering a dataset, forms unequal clusters. This metric leads to a larger size for a cluster with a centroid away from the origin, rather than a cluster closer to the origin. The proposed metric is based on the Canberra distances and it is …Deep Learning (DL) has shown great promise in the unsupervised task of clustering. That said, while in classical (i.e., non-deep) clustering the benefits of the nonparametric approach are well known, most deep-clustering methods are parametric: namely, they require a predefined and fixed number of clusters, denoted by K. When K is …Photo by Nathan Anderson @unsplash.com. In my last post of the Unsupervised Learning Series, we explored one of the most famous clustering methods, the K-means Clustering.In this post, we are going to discuss the methods behind another important clustering technique — hierarchical clustering! This method is also based on …Given that dealing with unlabelled data is one of the main use cases of unsupervised learning, we require some other metrics that evaluate clustering results without needing to refer to ‘true’ labels. Suppose we have the following results from three separate clustering analyses. Evidently, the ‘tighter’ we can make our clusters, the better.Hyperspectral images are becoming a valuable tool much used in agriculture, mineralogy, and so on. The challenge is to successfully classify the materials ...In the last blog, I had talked about how you can use Autoencoders to represent the given input to dense latent space. Here, we will see one of the classic algorithms thatGraph Clustering with Graph Neural Networks. Graph Neural Networks (GNNs) have achieved state-of-the-art results on many graph analysis tasks such as node classification and link prediction. However, important unsupervised problems on graphs, such as graph clustering, have proved more resistant to advances in GNNs.Use the following steps to access unsupervised machine learning in DSS: Go to the Flow for your project. Click on the dataset you want to use. Select the Lab. Create a new visual analysis. Click on the Models tab. Select Create first model. Select AutoML Clustering.Unsupervised landmarks discovery (ULD) for an object category is a challenging computer vision problem. In pursuit of developing a robust ULD framework, …Clustering. Clustering, an application of unsupervised learning, lets you explore your data by grouping and identifying natural segments. Use clustering to explore clusters generated from many types of data—numeric, categorical, text, image, and geospatial data—independently or combined. In clustering mode, DataRobot captures a …Unsupervised domain adaptation (UDA) is to make predictions for unlabeled data on a target domain, given labeled data on a source domain whose distribution shifts from the target one. Mainstream UDA methods learn aligned features between the two domains, such that a classifier trained on the source features can be readily applied to …Unsupervised learning algorithms need only X (features) without y (labels) to work, as they tend to find similarities in data and based on them conduct ...Next, under each of the X cluster nodes, the algorithm further divide the data into Y clusters based on feature A. The algorithm continues until all the features are used. The algorithm that I described above is like a decision-tree algorithm. But I need it for unsupervised clustering, instead of supervised classification.Families traveling with young children can soon score deep discounts on flights to the Azores. The Azores, a cluster of nine volcanic islands off the coast of Portugal, is one of t...Clustering is the most popular unsupervised learning algorithm; it groups data points into clusters based on their similarity. Because most datasets in the world are unlabeled, unsupervised learning algorithms are very applicable. Possible applications of clustering include: Search engines: grouping news topics and search results. Market ...Clustering is one of the most crucial problems in unsupervised learning, and the well-known k-means algorithm can be implemented on a quantum computer with a significant speedup.However, for the clustering problems that cannot be solved using the k-means algorithm, a powerful method called spectral clustering is used.In this study, we …In this paper, we advocate an unsupervised learning approach to clustering pixels based on distinctive polarization features, which allows for identifying specific spatial organization via ...Unsupervised clustering models have been widely used for multimetric phenotyping of complex and heterogeneous diseases such as diabetes and obstructive sleep apnea (OSA) to more precisely ...In today’s digital age, automotive technology has advanced significantly. One such advancement is the use of electronic clusters in vehicles. A cluster repair service refers to the...Cluster headache pain can be triggered by alcohol. Learn more about cluster headaches and alcohol from Discovery Health. Advertisement Alcohol can trigger either a migraine or a cl...Distinguishing useful microseismic signals is a critical step in microseismic monitoring. Here, we present the time series contrastive clustering (TSCC) method, an end-to-end unsupervised model for clustering microseismic signals that uses a contrastive learning network and a centroidal-based clustering model. The TSCC framework consists of two …Advertisement Deep-sky objects include multiple stars, variable stars, star clusters, nebulae and galaxies. A catalog of more than 100 deep-sky objects that you can see in a small ...Learn about clustering methods, such as k-means and hierarchical clustering, and dimensionality reduction, such as PCA. See examples, algorithms, pros and cons, and …Unsupervised clustering is of central importance for the analysis of these data, as it is used to identify putative cell types. However, there are many challenges …Unsupervised clustering reveals clusters of learners with differing online engagement. To find groups of learners with similar online engagement in an unsupervised manner, we follow the procedure ...Unsupervised learning is a type of machine learning algorithm used to draw inferences from datasets without human intervention, in contrast to supervised learning where labels are provided along with the data. The most common unsupervised learning method is cluster analysis, which applies clustering methods to explore data and find hidden ...Time Series Clustering is an unsupervised data mining technique for organizing data points into groups based on their similarity. The objective is to maximize data similarity within clusters and minimize it across clusters. The project has 2 parts — temporal clustering and spatial clustering.I have an unsupervised K-Means clustering model output (as shown in the first photo below) and then I clustered my data using the actual classifications. The photo below are the actual classifications. I am trying to test, in Python, how well my K-Means classification (above) did against the actual classification. ...Some plants need a little more support than the rest, either because of heavy clusters of flowers or slender stems. Learn about staking plants. Advertisement Some plants need just ...The CCST framework. We extended the unsupervised node embedding method Deep Graph Infomax (DGI) 36 and developed CCST to discover cell subpopulations from spatial single-cell expression data. As ...To overcome the shortcomings of the existing approaches, we introduce a new algorithm for key frame extraction based on unsupervised clustering. The proposed algorithm is both computationally simple and able to adapt to the visual content. The efficiency and effectiveness are validated by large amount of real-world videos. ...In unsupervised learning, the machine is trained on a set of unlabeled data, which means that the input data is not paired with the desired output. The machine then learns to find patterns and relationships in the data. Unsupervised learning is often used for tasks such as clustering, dimensionality reduction, and anomaly detection.Unsupervised nearest neighbors is the foundation of many other learning methods, notably manifold learning and spectral clustering. Supervised neighbors-based learning comes in two flavors: classification for data with discrete labels, and regression for data with continuous labels.Clustering algorithms form groupings in such a way that data within a group (or cluster) have a higher measure of similarity than data in any other cluster. Various similarity measures can be used, including Euclidean, probabilistic, cosine distance, and correlation. Most unsupervised learning methods are a form of cluster analysis.Some plants need a little more support than the rest, either because of heavy clusters of flowers or slender stems. Learn about staking plants. Advertisement Some plants need just ...There are two common unsupervised ways to build tasks from the auxiliary dataset: 1) CSS-based methods (Comparative Self-Supervised, as shown in Fig. 1(c)) use data augmentations to obtain another view of the images to construct the image pairs, and then use the image pairs to build tasks [17, 20]; 2) Clustering-based methods (as shown …Earth star plants quickly form clusters of plants that remain small enough to be planted in dish gardens or terrariums. Learn more at HowStuffWorks. Advertisement Earth star plant ...Clustering: Clustering is the process of grouping similar data points, it is an unsupervised Machine Learning technique, and the main goal of an unsupervised ML technique is to find similarities ...Distinguishing useful microseismic signals is a critical step in microseismic monitoring. Here, we present the time series contrastive clustering (TSCC) method, an end-to-end unsupervised model for clustering microseismic signals that uses a contrastive learning network and a centroidal-based clustering model. The TSCC framework consists of two …Hierarchical clustering. Algorithm It is a clustering algorithm with an agglomerative hierarchical approach that build nested clusters in a successive manner. Types There are different sorts of hierarchical clustering algorithms that aims at optimizing different objective functions, which is summed up in the table below:In this paper, we therefore propose an unsupervised Bayesian clustering method termed Clustering 16S rRNA for OTU Prediction (CROP), which specifically addresses the problems of OTU overestimation, computational efficiency and memory requirement. This Bayesian method, if modeled properly, can infer the optimal clustering …To resolve this dilemma, we propose the FOrensic ContrAstive cLustering (FOCAL) method, a novel, simple yet very effective paradigm based on contrastive learning and unsupervised clustering for the image forgery detection. Specifically, FOCAL 1) utilizes pixel-level contrastive learning to supervise the high-level forensic feature extraction in ...Clustering is a classical unsupervised machine learning problem and has been studied extensively in recent decades. Many popular methods have been proposed, such as k-means 3 , Gaussian mixture ...Clustering is an unsupervised learning method having models – KMeans, hierarchical clustering, DBSCAN, etc. Visual representation of clusters shows the data in an easily understandable format as it groups elements of a large dataset according to their similarities. This makes analysis easy.Use the following steps to access unsupervised machine learning in DSS: Go to the Flow for your project. Click on the dataset you want to use. Select the Lab. Create a new visual analysis. Click on the Models tab. Select Create first model. Select AutoML Clustering.Unsupervised clustering is widely applied in single-cell RNA-sequencing (scRNA-seq) workflows. The goal is to detect distinct cell populations that can be annotated as known cell types or ...Given that dealing with unlabelled data is one of the main use cases of unsupervised learning, we require some other metrics that evaluate clustering results without needing to refer to ‘true’ labels. Suppose we have the following results from three separate clustering analyses. Evidently, the ‘tighter’ we can make our clusters, the better.Unsupervised Clustering of Southern Ocean Argo Float Temperature Profiles. Daniel C. Jones, Corresponding Author. Daniel C. Jones [email protected] ... GMM is a generalization of k-means clustering, which only attempts to minimize in-group variance by shifting the means. By contrast, GMM attempts to identify means and standard …GibbsCluster is a powerful tool for unsupervised motif discovery because it can simultaneously cluster and align peptide data. The GibbsCluster 2.0 presented here is an improved version incorporating insertion and deletions accounting for variations in motif length in the peptide input. In basic terms, the program takes as input a set of ...Looking for an easy way to stitch together a cluster of photos you took of that great vacation scene? MagToo, a free online panorama-sharing service, offers a free online tool to c...Unsupervised clustering is perhaps one of the most important tasks of unsupervised machine learning algorithms currently, due to a variety of application needs and connections with other problems. Clustering can be formulated as follows. Consider a dataset that is composed of N samples ...In this paper, we therefore propose an unsupervised Bayesian clustering method termed Clustering 16S rRNA for OTU Prediction (CROP), which specifically addresses the problems of OTU overestimation, computational efficiency and memory requirement. This Bayesian method, if modeled properly, can infer the optimal clustering …Given that dealing with unlabelled data is one of the main use cases of unsupervised learning, we require some other metrics that evaluate clustering results without needing to refer to ‘true’ labels. Suppose we have the following results from three separate clustering analyses. Evidently, the ‘tighter’ we can make our clusters, the better.Mailbox cluster box units are an essential feature for multi-family communities. These units provide numerous benefits that enhance the convenience and security of mail delivery fo...14. Check out the DBSCAN algorithm. It clusters based on local density of vectors, i.e. they must not be more than some ε distance apart, and can determine the number of clusters automatically. It also considers outliers, i.e. points with an unsufficient number of ε -neighbors, to not be part of a cluster.The commonly used unsupervised learning technique is cluster analysis, which is massively utilized for exploratory data analysis to determine the hidden …Hierarchical clustering. Algorithm It is a clustering algorithm with an agglomerative hierarchical approach that build nested clusters in a successive manner. Types There are different sorts of hierarchical clustering algorithms that aims at optimizing different objective functions, which is summed up in the table below:K-means is one of the simplest unsupervised learning algorithms that solve the well known clustering problem. The procedure follows a simple and easy way to classify a given data set through a certain number of clusters (assume k clusters) fixed a priori. The main idea is to define k centroids, one for each cluster.Clustering is a classical unsupervised machine learning problem and has been studied extensively in recent decades. Many popular methods have been proposed, such as k-means 3 , Gaussian mixture ...The Secret Service has two main missions: protecting the president and combating counterfeiting. Learn the secrets of the Secret Service at HowStuffWorks. Advertisement You've seen...This repository is the official implementation of PiCIE: Unsupervised Semantic Segmentation using Invariance and Equivariance in Clustering, CVPR 2021. Contact: Jang Hyun Cho [email protected] .Unsupervised image clustering. The primary purpose of UIC is to assign similar images to the same group. Since DNNs achieve superior performance for machine vision tasks [22], deep image clustering approaches tend to utilize DNNs to perform this task. However, the similarity of visual features across different semantic classes often …For visualization purposes we can reduce the data to 2-dimensions using UMAP. When we cluster the data in high dimensions we can visualize the result of that clustering. First, however, we’ll view the data colored by the digit that each data point represents – we’ll use a different color for each digit. This will help frame what follows.A parametric test is used on parametric data, while non-parametric data is examined with a non-parametric test. Parametric data is data that clusters around a particular point, wit...Introduction. K-means clustering is an unsupervised algorithm that groups unlabelled data into different clusters. The K in its title represents the number of clusters that will be created. This is something …Mailbox cluster box units are an essential feature for multi-family communities. These units provide numerous benefits that enhance the convenience and security of mail delivery fo...K-means doesn't allow noisy data, while hierarchical clustering can directly use the noisy dataset for clustering. t-SNE Clustering. One of the unsupervised learning methods for visualization is t-distributed stochastic neighbor embedding, or t-SNE. It maps high-dimensional space into a two or three-dimensional space which can then be visualized.Then, an unsupervised cluster method is used to produce dense regions. Each adjusted dense region is fed into the detector for object detection. Finally, a global merge module generates the final predict results. Experiments were conducted on two popular aerial image datasets including VisDrone2019 and UAVDT. In both datasets, our proposed ...K-means doesn't allow noisy data, while hierarchical clustering can directly use the noisy dataset for clustering. t-SNE Clustering. One of the unsupervised learning methods for visualization is t-distributed stochastic neighbor embedding, or t-SNE. It maps high-dimensional space into a two or three-dimensional space which can then be visualized.Cluster analysis is a staple of unsupervised machine learning and data science.. It is very useful for data mining and big data because it automatically finds patterns in the data, without the need for labels, unlike supervised machine learning.. In a real-world environment, you can imagine that a robot or an artificial intelligence won’t always have …01-Feb-2021 ... Check membership Perks: https://www.youtube.com/channel/UCG04dVOTmbRYPY1wvshBVDQ/join . This video is about Unsupervised Learning and the ...K-Means clustering is an unsupervised machine learning algorithm that is used to solve clustering problems. The goal of this algorithm is to find groups or clusters in the data, … Clustering: grouping observations together¶ The problem solved in clustering. Given the iris dataset, if we knew that there were 3 types of iris, but did not have access to a taxonomist to label them: we could try a clustering task: split the observations into well-separated group called clusters. . In today’s fast-paced world, security and convenience are two factorsClustering, the process of grouping together simil The places where women actually make more than men for comparable work are all clustered in the Northeast. By clicking "TRY IT", I agree to receive newsletters and promotions from ...Unsupervised clustering reveals clusters of learners with differing online engagement. To find groups of learners with similar online engagement in an unsupervised manner, we follow the procedure ... Want to know how to make a schedule for kids after-school? Visit Ho The places where women actually make more than men for comparable work are all clustered in the Northeast. By clicking "TRY IT", I agree to receive newsletters and promotions from ... Clustering methods. There are three main clustering ...
Continue Reading